Tech
Inexpensive Microplastic Monitoring Through Porous Materials and Machine Learning
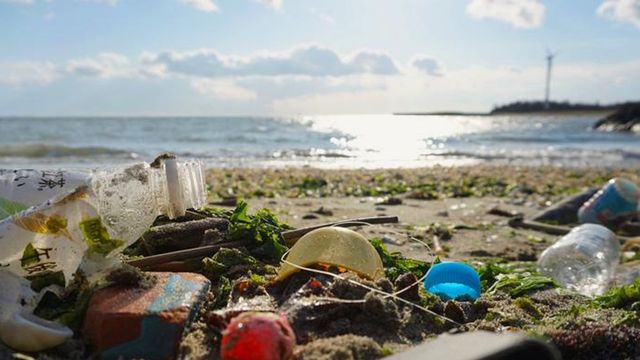
Thank you. Listen to this article using the player above. ✖
Want to listen to this article for FREE?
Complete the form below to unlock access to ALL audio articles.
Optical analysis and machine learning techniques can now readily detect microplastics in marine and freshwater environments using inexpensive porous metal substrates. Details of the method, developed by researchers at Nagoya University with collaborators at the National Institute for Materials Sciences in Japan and others, are published in the journal Nature Communications.
Detecting and identifying microplastics in water samples is essential for environmental monitoring but is challenging due in part to the structural similarity of microplastics with natural organic compounds derived from biofilms, algae, and decaying organic matter. Existing detection methods generally require complex separation techniques that are time-consuming and costly.
Want more breaking news?
Subscribe to Technology Networks’ daily newsletter, delivering breaking science news straight to your inbox every day.
“Our new method can simultaneously separate and measure the abundance of six key types of microplastics – polystyrene, polyethylene, polymethylmethacrylate, polytetrafluoroethylene, nylon and polyethylene terephthalate,” says Dr. Olga Guselnikova of the National Institute for Materials Science (NIMS).
The system uses a porous metal foam to capture microplastics from solution and detect them optically using a process called surface-enhanced Raman spectroscopy (SERS). “The SERS data obtained is highly complex,” explains Dr. Joel Henzie of NIMS, “but it contains discernible patterns that can be interpreted using modern machine learning techniques.”
To analyse the data, the team created a neural network computer algorithm called SpecATNet. This algorithm learns how to interpret the patterns in the optical measurements to identify the target microplastics more quickly and with higher accuracy than traditional methods.
“Our procedure holds immense potential for monitoring microplastics in samples obtained directly from the environment, with no pretreatment required, while being unaffected by possible contaminants that could interfere with other methods,” says Professor Yusuke Yamauchi of Nagoya University.
The researchers hope their innovation will greatly assist society in evaluating the significance of microplastic pollution on public health and the health of all organisms in marine and freshwater environments. By creating inexpensive microplastic sensors and open-source algorithms to interpret data, they hope to enable the rapid detection of microplastics, even in resource-limited labs.
Currently, materials required for the new system bring cost savings of 90 to 95% compared to commercially available alternatives. The group plans to drive the cost of these sensors down even further and make the methods simple to replicate without the need for expensive facilities. In addition, the researchers hope to expand the capability of the SpecATNet neural network to detect a broader range of microplastics and even accept different kinds of spectroscopic data in addition to SERS data.
Reference: Guselnikova O, Trelin A, Kang Y, et al. Pretreatment-free SERS sensing of microplastics using a self-attention-based neural network on hierarchically porous Ag foams. Nat Comm. 2024;15(1):4351. doi: 10.1038/s41467-024-48148-w
This article has been republished from the following materials. Note: material may have been edited for length and content. For further information, please contact the cited source. Our press release publishing policy can be accessed here.