Fitness
From ‘CyberSlug’ to ‘CyberOctopus’: New AI explores, remembers, seeks novelty, overcomes obstacles
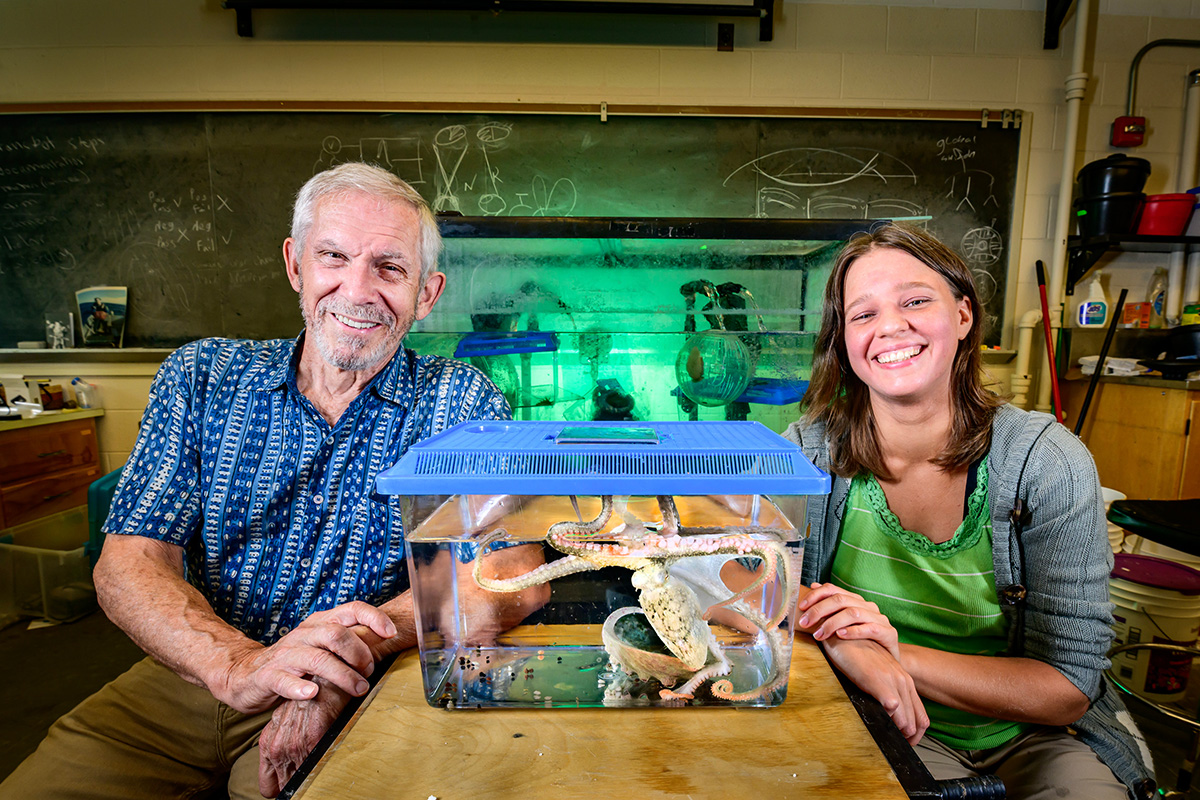
CHAMPAIGN, Ill. — By giving artificial intelligence simple associative learning rules based on the brain circuits that allow a sea slug to forage — and augmenting it with better episodic memory, like that of an octopus — scientists have built an AI that can navigate new environments, seek rewards, map landmarks and overcome obstacles.
Reported in the journal Neurocomputing, the new approach gives AI the ability to explore and gather the information it needs to expand its spatial and temporal awareness, growing its knowledge base while learning on the job, said Ekaterina Gribkova, a postdoctoral researcher at the University of Illinois Urbana-Champaign who led the study with U. of I. molecular and integrative physiology professor emeritus Rhanor Gillette, with support from agricultural and biological engineering professor Girish Chowdhary.
“This essentially makes our artificial intelligence much more animal-like than current artificial intelligences,” Gribkova said. “We are learning how to go from something like the memory of a sea slug, which is very, very simple, to something like us.”
The new approach has the added advantage of being more efficient than the standard method of pretraining AI with vast amounts of data before it performs basic functions, she said.
By adding a memory module that allows the AI to retain information about past events, very simple spatial learning can be expanded to much more complex learning, Gribkova said.
“We can use the same types of associations to learn, for example, sequences of motor behaviors, or how to map social networks or even linguistic problem-solving,” she said. “Learning in the spatial domain, and actually navigating the environment making shortcuts, which our memory module is able to do, can be very well applied to more abstract concepts.”
To achieve this, the team built on their previous work simulating the decision-making neural circuits of a sea slug of the genus Pleurobranchaea, a project known as “Cyberslug.” They named their simulated creature ASIMOV, after Isaac Asimov, a science fiction writer who explored the safety concerns and ethics of robotics in human society.
The researchers programmed ASIMOV to monitor its own internal state and seek satiation and reward. When it was “hungry,” after not having eaten for a while, ASIMOV learned through trial and error to select nutritious over noxious food items. When the researchers added an intoxicating simulated drug to its range of options, ASIMOV behaved as if addicted, chasing after the drug even though it had no nutritional value.
ASIMOV lived in the moment, however, and while it could learn from experience, its memory and ability to integrate information from past experiences were limited. So Gribkova added a computational module for episodic memory, called the Feature Association Matrix, modeled on the architecture and functions of the hippocampus, a brain region essential to learning and memory. This work also was informed by studies of the brain networks that drive behavior in an octopus. The researchers called the ASIMOV-FAM agent a “CyberOctopus.”
“Episodic memory encodes both spatial and temporal contexts of past events and experience,” the authors wrote. This is a fundamental building block of natural intelligence, which most current AI models lack, they said.
In the new study, ASIMOV-FAM explored a simulated environment with various landmarks, some of which had rewards attached to them. Gribkova focused on pairwise associations that could be strengthened or weakened by weighing the relative rewards of each pathway ASIMOV took. It was programmed to seek out novelty and rewards.
“The ASIMOV agent uses cognitive maps formed by the FAM to learn its spatial environment,” Gillette said. “It can generate new paths and shortcuts for traversing the environment more efficiently, for greater rewards. This is effectively spatial reasoning.”
The researchers said they expect to use this new approach to create a more efficient and advanced AI that can perform a variety of tasks beyond spatial navigation.
“We expect ASIMOV-FAM can be adapted for more abstract, nonspatial applications, for instance, to enhance large language models like ChatGPT for more efficient computation and problem-solving, with reduced size and training requirements,” Gillette said.
“We’re thinking that if we can do more of a bottom-up approach, we can build an AI that doesn’t require all that much data, that’s a lot more animal-like in its adaptive behavior and creativity and that is learning more on its own,” Gribkova said. “In essence, we see advanced AI as learning much more like a child would learn.”
Gillette and Gribkova are affiliates of the Center for Artificial Intelligence Innovation at the National Center for Supercomputing Applications and in the Neuroscience Program at the U. of I. Gillette also is a professor in the Beckman Institute for Advanced Science and Technology and in the Carl R. Woese Institute for Genomic Biology at Illinois.
The U.S. Office of Naval Research supported this work.